Are you exhausted from constantly prompting engineering tasks? Do you find the process fragile and tiresome? Are you involved in creating workflows using language models? If so, you might be interested in DSPy. This blog post provides a gentle introduction to its core concepts.
While building robust neuro-symbolic AI workflows, we’ll explore the synergy between LMs and graph knowledge bases within digital marketing and SEO tasks.
Table of content:
- What is DSPy?
- Let’s Build Our First Agents
- Automated Optimization Using DSPy Compiler
- Creating a Learning Agent
- Implementing Multi-Hop Search with DSPy and WordLift
- Conclusion and Future Work
What is DSPy?
DSPy is an acronym that stands for Declarative Self-Improving Language Programs. It is a framework developed by the Stanford NLP team that aims to shift the focus from using LMs with orchestrating frameworks like LangChain, Llama Index, or Semantic Kernel to programming with foundational models. This approach addresses the need for structured and programming-first prompting that can improve itself over time.
A Real Machine Learning Workflow🤩
For those with experience working with PyTorch and machine learning in general, DSPy is an excellent tool. It is designed around the concept of constructing neural networks. Let me explain: when starting a machine learning project, you typically begin by working on datasets, defining the model, running the training, configuring the evaluation, and testing.
DSPy simplifies this process by providing general-purpose modules like ChainOfThought and ReAct, which you can use instead of complex prompting structures. Most importantly, DSPy brings general optimizers (BootstrapFewShotWithRandomSearch or BayesianSignatureOptimizer), which are algorithms that will automatically update the parameters in your AI program.
You can recompile your program whenever you change your code, data, assertions, or metrics, and DSPy will generate new effective prompts that fit your modifications.
DSPy’s design philosophy is the opposite of thinking, “Prompting is the future of programming.” you will build modules to express the logic behind your task and let the framework deal with the language model.
Core Concepts Behind DSPy💡
Let’s review the fundamental components of the framework:
- Signatures: Here is how you abstract both prompting and fine-tuning. Imagine signatures as the core directive of your program (e.g., read a question and answer it, do sentiment analysis, optimize title tags). This is where you define the inputs and outputs of your program; it’s the contract between you and the LM. Question answering is represented, for example as question -> answer, sentiment analysis is sentence -> sentiment, title optimization is title, keyword -> optimized title and so on.
- Modules: They are the building blocks of your program. Here is where you define how things shall be done (e.g., use Chain of Thought or Act as an SEO specialist, etc.). Using parameters here, you can encode your prompting strategies without directly messing up with prompt engineering. DSPy comes with pre-defined modules (dspy.Predict, dspy.ChainOfThought, dspy.ProgramOfThought, dspy.ReAct, and so on ). Modules use the Signature as an indication of what needs to be done.
- Optimizers: to improve the accuracy of the LM, a DSPy Optimizer will automatically update the prompts or even the LM’s weights to improve on a given metric. To use an optimizer, you will need the following:
- a DSPy program (like a simple dspy.Predict or a RAG),
- a metric to assess the quality of the output,
- a training dataset to identify valuable examples (even small batches like 10 or 20 would work here).
Let’s Build Our First Agents 🤖
Before further ado, let’s begin with a few examples to dive into the implementation by following a simple Colab Notebook.
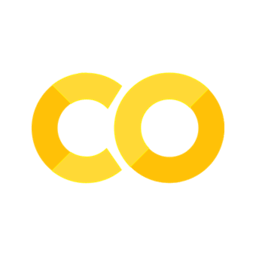
We will:
- Run a few basic examples for zero-shot prompting, entity extraction, and summarization. These are simple NLP tasks that we can run using LMs. We will execute them using DSPy to grasp its intrinsic logic.
- After familiarizing ourselves with these concepts, we will implement our first Retrieval-Augmented Generation (RAG) pipeline. Retrieval-augmented generation (RAG) allows LMs to tap into a large corpus of knowledge from sources such as a Knowledge Graph (KG). The RAG will query the KG behind this blog to find the relevant passages/content that can produce a well-refined response. Here, we will construct a DSPy retriever using WordLift’s Vector Search. It is our first time using this new functionality from our platform 😍.
- We will then:
a. Compile a program using the RAG previously created.
b. Create a training dataset by extracting question-answer pairs from our KG (we will extract a set of schema:faqPages).
c. Configure a DSPy Optimizer to improve our program.
d. Evaluate the results.
A Simple Workflow For Content Summarization🗜️
Let’s create a signature to elaborate a summary from a long text. This can be handy for generating the meta description of blog posts or other similar tasks. We will simply instruct DSPy to use long_context -> tldr using the Chain Of Thought methodology.
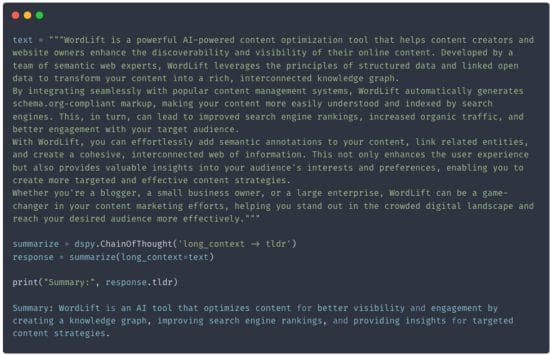
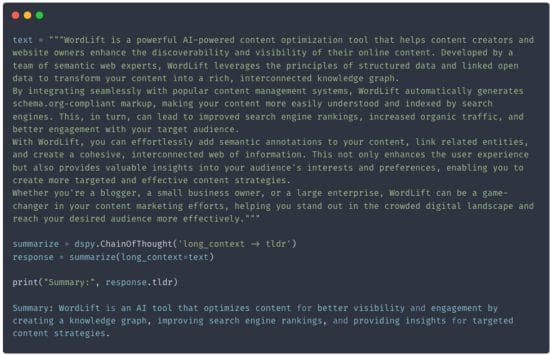
Worth noticing we didn’t have to write any prompts!
WordLift DSPy Retriever🔎
The next step is to use WordLift’s Knowledge Graph and its new semantic search capabilities. DSPy supports various retrieval modules out of the box, such as ColBERTv2, AzureCognitiveSearch, Pinecone, Weaviate, and now also WordLift 😎.
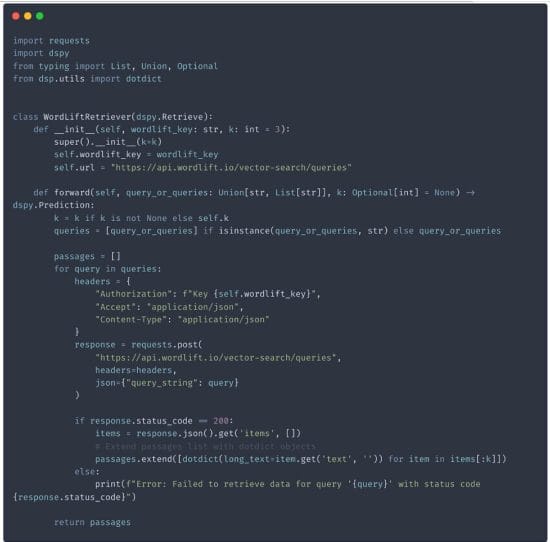
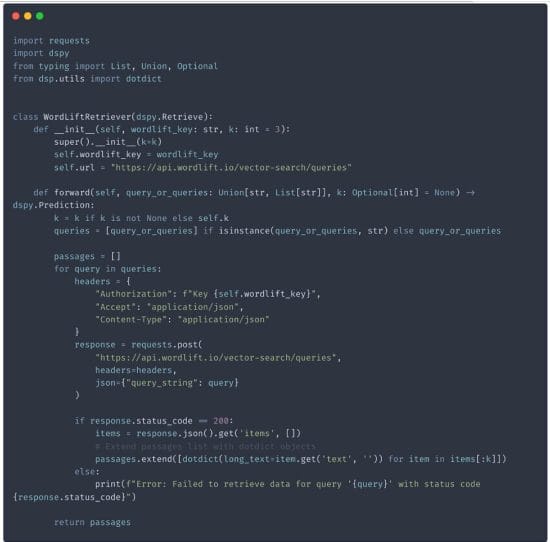
Here is how we’re creating the WordLiftRetriever, which, given a query, will provide the most relevant passages.
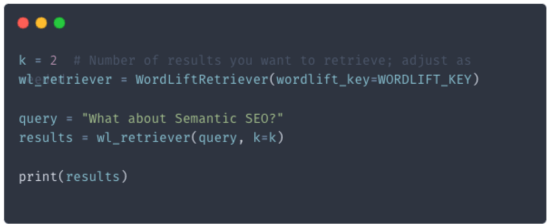
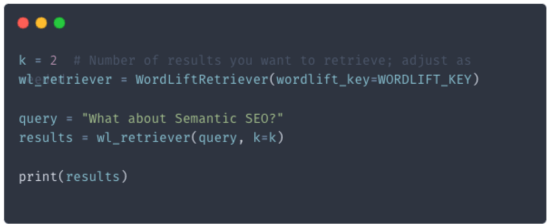
Building a RAG, once we have a retriever, using DSPy is quite straightforward. We begin by setting up both the language model and the new retriever with the following line:
dspy.settings.configure(lm=turbo, rm=wl_retriever)
The RAG comprises a signature made of a context (obtained from WordLift’s KG), a question, and an answer.
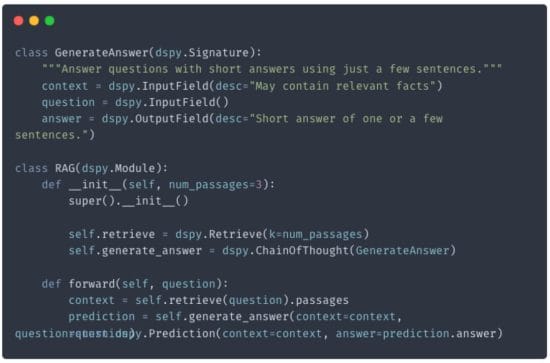
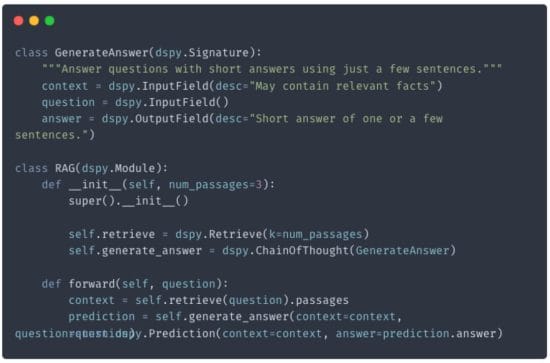
Automated Optimization Using DSPy Compiler
Using the DSPy compiler, we can now optimize the performance or efficiency of an NLP pipeline by simulating different program versions and bootstrapping examples to construct effective few-shot prompts.
I like this about DSPy: not only are we moving away from chaining tasks, but we’re using programming and can rely on the framework to automate the prompt optimization process.
The “optimizer” component, previously known as the teleprompter, helps to refine a program’s modules by prompting or fine-tuning the optimization process. This is the real magic of using the DSPy framework. As we feed more data into our Knowledge Graph, the AI agent we create using DSPy evolves to align its generation with the gold standard we have established.
Let’s Create a DSPy Program
DSPy programs like the one built in the Colab help us with tasks like question answering, information extraction, or content optimization.
As with traditional machine learning, the general workflow comprises these steps:
- Get data. To train your program, you will need some training data. To do this, you should provide examples of the inputs and outputs that your program will use. For instance, collecting FAQs from your blog will give you a relevant set of question-answer pairs. Using at least 10 samples is recommended, but remember that the more data you have, the better your program will perform.
- Write your program. Define your program’s modules (i.e., sub-tasks) and how they should interact to solve your task. We are using primarily a RAG with a Chain Of Thought. Imagine using control flows if/then statements and effectively using the data in our knowledge base and external APIs to accomplish more sophisticated tasks.
- Define some validation logic. What makes for a good run of your program? Maybe the answers we have already marked up as FAQs? Maybe the best descriptions for our products? Specify the logic that will validate that.
- Compile! Ask DSPy to compile your program using your data. The compiler will use our data and validation logic to optimize the program (e.g., prompts and modules).
- Iterate. Repeat the process by improving your data, program, and validation or using more advanced DSPy compiler features.
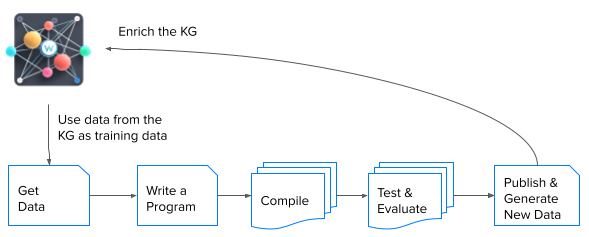
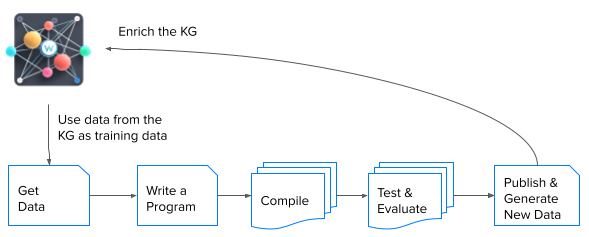
Creating a Learning Agent
By combining DSPy with curated data in a graph, we can create LM-based applications that are modular, easy to maintain, self-optimizing, and robust to changes in the underlying models and datasets. The synergies between semantic data and a declarative framework like DSPy enable a new paradigm of LLM programming, where high-level reasoning strategies (i.e., optimize the product description by reading all the latest reviews) can be automatically discovered, optimized, and integrated into efficient and interpretable pipelines.
DSPy is brilliant as it creates a new paradigm for AI agent development. Using the DSPy compiler we can ground the generation in the information we store in our knowledge graph and have a system that is self-optimizing and easier to be understood.
Here is DSPy’s teleprompter and compiler pipeline, which helps us create a modular, extensible, self-optimizing RAG system that adapts by leveraging human-annotated question-answer pairs on our website!
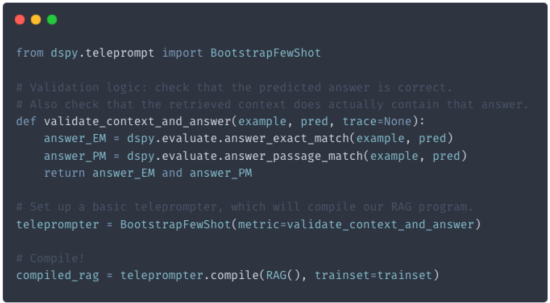
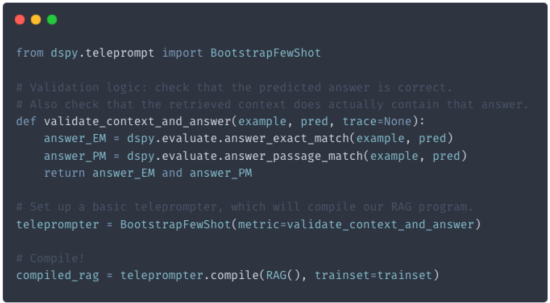
Implementing Multi-Hop Search with DSPy and WordLift
When dealing with complex queries that combine multiple information needs, we can implement a sophisticated retrieval mechanism, Multi-Hop Search (“Baleen” – Khattab et al., 2021), to help us find different parts of the same query in different documents.
Using DSPy, we can recreate such a system that will read the retrieved results and generate additional queries to gather further information when necessary.
We can do it with only a few lines of code.
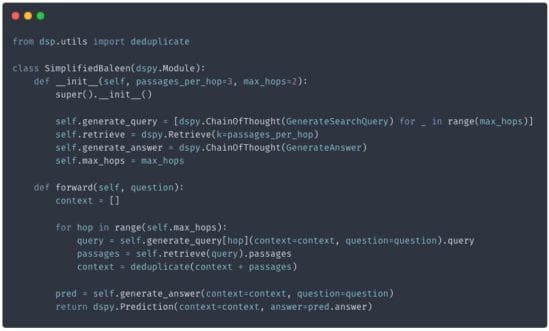
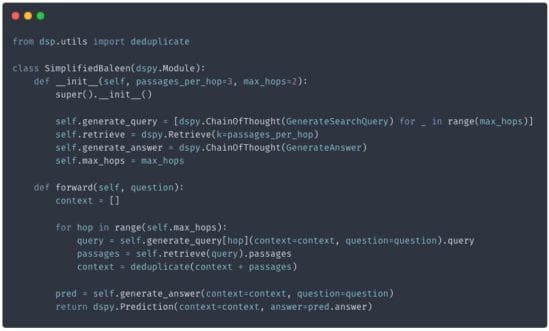
Let’s review this bare-bone implementation. The __init__ method defines a few key sub-modules:
- generate_query: We use the Chain of Thought predictor within the GenerateSearchQuery signature for each turn.
- retrieve: This module uses WordLift Vector Search to do the actual search using the generated queries.
- generate_answer: This dspy.Predict module is used after all the search steps. It has a GenerateAnswer to produce the final answer. The forward method uses these sub-modules in simple control flow. First, we’ll loop up to self.max_hops times. We generate a search query during each iteration using the predictor at self.generate_query[hop]. We’ll retrieve the top-k passages using that query. We’ll add the (deduplicated) passages to our accumulator of context. After the loop, we’ll use self.generate_answer to produce the final answer. We’ll return a prediction with the retrieved context and the predicted answer.
Quite interestingly, we can inspect the last calls to the LLM with a simple command: turbo.inspect_history(n=3). This is a practical way to examine the extensive optimization work done automatically with these very few lines of code.
Conclusion and Future Work
As new language models emerge with advanced abilities, there is a trend to move away from fine-tuning and towards more sophisticated prompting techniques.
The combination of symbolic reasoning enabled by function calling and semantic data requires a robust AI development and validation strategy.
While still at its earliest stage, DSPy represents a breakthrough in orchestration frameworks. It improves language programs with more refined semantic data, clean definitions, and a programming-first approach that best suits our neuro-symbolic thinking.
Diving deeper into DSPy will help us improve our tooling and Agent WordLift’s skills in providing more accurate responses. Evaluation in LLM applications remains a strategic goal, and DSPy brings the right approach to solving the problem.
Imagine the potential advancements in generating product descriptions as we continuously enrich the knowledge graph (KG) with additional training data. Integrating data from Google Search Console will allow us to pinpoint and leverage the most effective samples to improve a DSPy program.
Beyond SEOs and digital marketing, creating self-optimizing AI systems raises ethical implications for using these technologies. As we develop increasingly powerful and autonomous AI agents and workflows, it is vital that we do so responsibly and in a way that is fully aligned with human values.
Are you evaluating the integration of Generative AI within your organization to enhance marketing efforts? I am eager to hear your plans. Drop me a line with your thoughts.
References
#Meeting #DSPy #SEO #Programming #Framework